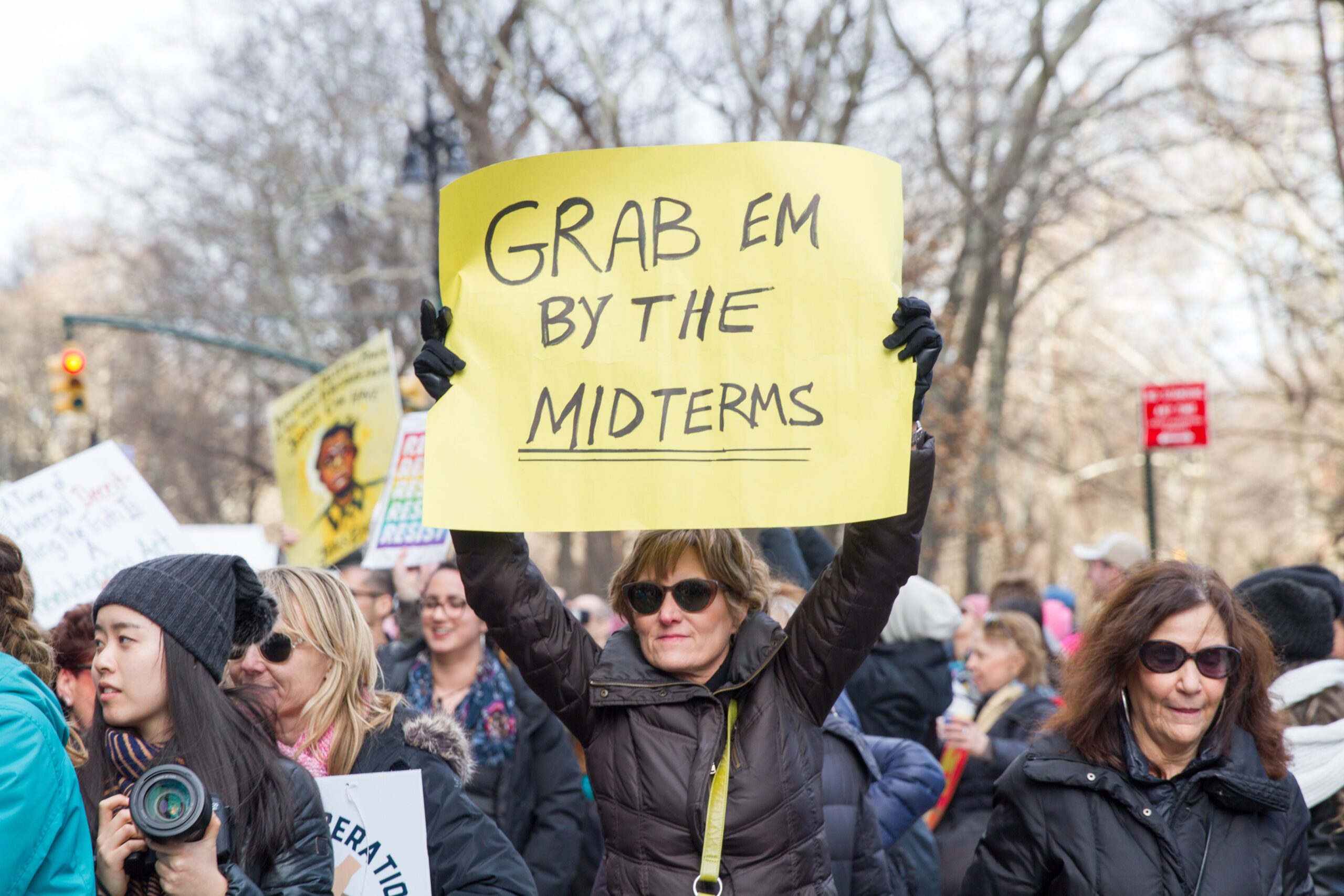
2018 Midterms: Did the polls fail—again?!
This post is part of an ongoing series on the ins and outs of polling. If you missed the previous posts on Five Things to Pay Attention to in a New Poll or Polling Underrepresented Communities, go back and read them! Our series will continue with posts from our comms colleagues on incorporating polls and public opinion into your media work, so stay tuned.
We keep asking this question!
Did the polls fail? How could the polls have been off by so much? Should we even trust polls anymore?!
In 2016, polling models (in)famously failed to predict a Trump victory. Many of us in the advocacy community were obsessively refreshing the FiveThirtyEight election model page and talking your friends off a ledge when it remained unlikely that Trump would win. Even Trump himself was surprised and unprepared for victory.
After Election Night 2016, the polling industry itself did some soul-searching. Among other effects, the “failure” of the polls prompted the Associated Press to leave the National Election Pool and form a competitor to the Exit Poll, which other media companies joined for 2018.
And this year, polls in Florida showed Andrew Gillum and Bill Nelson with the lead in their respective races going into November 6. Yet both recently conceded their races to their opponents.
So…did the polls fail us? In a word: no.
In 2016, the polls didn’t fail—the models did
Many people wrote extensively on this question in the aftermath of the 2016 election (and, in fact, leading up to it). If you’re interested in a deep dive, I suggest starting with FiveThirtyEight and going on from there. But the important points are these:
-
National polls aligned with the popular vote.
-
State polls were not as accurate. And because our presidential elections rest on a wacky, unfair electoral college system in which some states have massively outsized power, a small inaccuracy in several key states obscured the likelihood of a Trump victory.
-
It wasn’t polls that failed, it was the models. Polls themselves are tools to measure the present. It’s election models that try to use current information to predict the future, and that is where we went rather wrong.
Given these three factors, it’s worth distinguishing between polls and models.
OK, but the models are based on polls, right?
Polls are good at finding out what big populations think. But polls are not as good at predicting the future.
Remember that polls are trying to find out about a universe of people by taking a small sample of those people. That universe can be all American adults, or people of color in a state, or people who frequently shop at Target. The idea is the same: take a large enough sample of that universe and you can tell a lot about it without having to ask every person in that universe.
Election polls—that is, polls asking about voters’ choices in an upcoming election—are trying to survey a group that doesn’t yet exist: voters. Up to the point when voters literally cast their ballots, everything is probability and supposition. We are supposing that some people will actually vote, and supposing others will not. As Ariel Edwards-Levy from the Huffington Post put it, “Today [on Election Day], across the nation, we’re seeing that universe be created, person by person.”
Polling tells you what is true now. Even if a pollster guesses correctly about who is likely to vote, all we know is what the outcome would (probably) be if the election were held today and those people did vote. We are assuming that what was true when the poll was taken will be true on November 6.
Polls already have blind spots and error: no sample perfectly represents a population, and many polls do a poor job of accounting for certain groups of people. When you add in the uncertainty of a population that doesn’t yet exist (people who will actually vote in the upcoming election), the chances of getting it wrong go up. Pollsters make educated guesses about who is in that universe of “likely voters” and who isn’t. They base these guesses on a lot of elements—the most important is whether someone voted in a previous election—but there are a lot of things that pollsters can’t foresee.
For example, a confusing ballot design in Broward County, FL. Or voting machines miscounting ballots in some Florida counties. Or problems with the design, not of the ballot itself, but of the return envelope for absentee ballots, as we saw in Gwinnett County, GA. Apart from election administration issues like these, tropical storms and other natural disasters can interfere with voter registration deadlinesrain on Election Day can make it hard for voters to wait in long lines at polling places—and wet ballots can cause malfunctions.
There are a lot of things that pollsters simply can’t account for when drawing and weighting their samples of “likely voters”—things that go well beyond an October surprise.
But the models are complex, scientific algorithms—right?!
It’s true that election prediction models like the one from FiveThirtyEight and The Upshot are complex. They “ingest” a lot of information, from national and state polls to fundraising levels, historical trends in turnout, and even experts’ ratings. Normally, we endorse the idea behind these models: rather than relying on a single poll, compare a bunch of polls and use additional relevant information to find the “truthiest” truth.
However, there are some potential problems that these models have a hard time dealing with. “Herding” is one problem Nate Silver has written about—basically, pollsters aren’t weighting their samples in a vacuum, but may be making their results look similar to their colleagues’ or like they think they “should” look. When pollsters are listening too closely to “conventional wisdom,” their polls may start to look like their expectations rather than reflecting the current population of voters they’re trying to sample.
Similarly, pollsters and model-builders make assumptions about the future based on the past, but some of those assumptions may be wrong. For example, Americans of color vote at lower rates, and less consistently, than white Americans. Pollsters will weight their samples accordingly, and models will also assume that respondents of color are less likely to turn out. But Black voters have voted at nearly the same rates as white voters in the last few elections, and many advocates are trying to change this trend for all racial groups. If the models do not account for this—or if they overcompensate—then they may be off.
Also related are systemic problems and diminishing returns. As Silver points out, “it’s better to be ahead in two polls than ahead in one poll, and in 10 polls than in two polls. Before long, however, you start to encounter diminishing returns. Polls tend to replicate one another’s mistakes.” In 2016, most polls apparently missed the group of white, male voters without college degrees who made a big difference to Trump’s win. Across most polling, it’s harder to get people of color to participate in a survey than white people, so polls systematically “miss” these groups. Adding another 10 or 20 polls to a model won’t make it more accurate if there is a systemic flaw.
Finally, remember that turnout models—like much of the polling world—are more of an art using scientific approaches than a true science. Each aspect of a model is a decision made by a person, who has biases and blind spots like any other person.
Bottom line: is polling broken?
Elections are hard to call because they’re make-believe until they’re not. Past may be prologue, but it isn’t prescription.
Polls will never be perfect. They tell us about the present, but they can’t see clearly into the future. They can’t account for poor ballot design, machine malfunction, weather, or the myriad other factors that may affect who votes and how.
In 2018, the polls seemed to be more accurate than not, as they have been for several years. Late polls showing Gillum and Nelson winning their contests when they both ended up losing is not a sign that polls are untrustworthy, but may point to other phenomena like herding or unforeseeable elements.
The extent to which you trust polls should also depend on what you’re using them for. Most of us use polls to read public opinion, not future behavior. For this purpose, the precision of the win-loss percentage is less crucial. What’s more important is that we know who thinks what, how that has changed over time, and what we can do about it.
To that end, the next posts in our public opinion series will be about how to use polls in your media work, from using social norming and FOMO to creating a compelling narrative for specific audiences.
If you have questions about polling methodology, the election, or public opinion, write to us at analysis@rethinkmedia.org.